Nonlinear Filtering and Smoothing: An Instroduction to Martingales, Stochastic Integrals and Estimation 1st edition by Venkatarama Krishnan – Ebook PDF Instant Download/Delivery. B00FC1N1KS 978-0486441641
Full download Nonlinear Filtering and Smoothing: An Instroduction to Martingales, Stochastic Integrals and Estimation 1st editon after payment
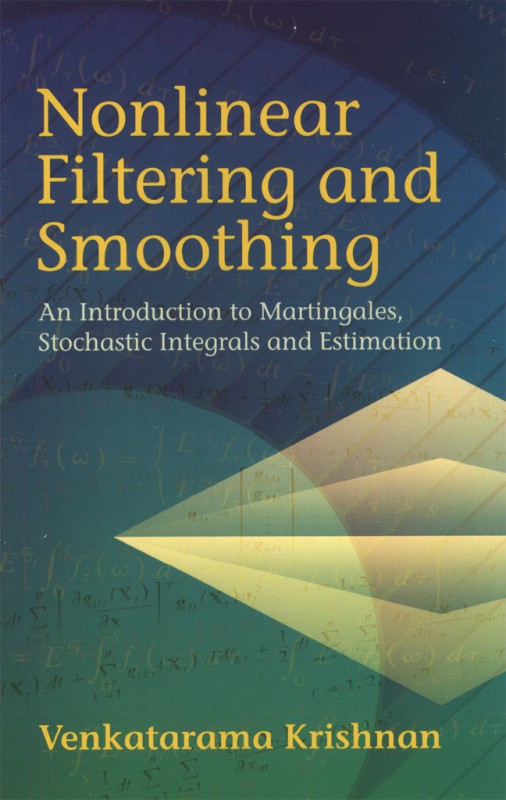
Product details:
ISBN 10: B00FC1N1KS
ISBN 13: 978-0486441641
Author: Venkatarama Krishnan
Most useful for graduate students in engineering and finance who have a basic knowledge of probability theory, this volume is designed to give a concise understanding of martingales, stochastic integrals, and estimation. It emphasizes applications. Many theorems feature heuristic proofs; others include rigorous proofs to reinforce physical understanding. Numerous end-of-chapter problems enhance the book’s practical value.
After introducing the basic measure-theoretic concepts of probability and stochastic processes, the text examines martingales, square integrable martingales, and stopping times. Considerations of white noise and white-noise integrals are followed by examinations of stochastic integrals and stochastic differential equations, as well as the associated Ito calculus and its extensions. After defining the Stratonovich integral, the text derives the correction terms needed for computational purposes to convert the Ito stochastic differential equation to the Stratonovich form. Additional chapters contain the derivation of the optimal nonlinear filtering representation, discuss how the Kalman filter stands as a special case of the general nonlinear filtering representation, apply the nonlinear filtering representations to a class of fault-detection problems, and discuss several optimal smoothing representations.
Nonlinear Filtering and Smoothing: An Instroduction to Martingales, Stochastic Integrals and Estimation 1st Table of contents:
-
Introduction to Stochastic Processes
- 1.1. Overview of Stochastic Processes
- 1.2. Random Variables and Expectation
- 1.3. Brownian Motion and Wiener Process
- 1.4. Markov Processes
- 1.5. Stochastic Integrals and Their Properties
-
Chapter 1: Basics of Filtering and Estimation
- 2.1. Introduction to Estimation Theory
- 2.2. Linear vs Nonlinear Estimation
- 2.3. State-Space Models for Filtering
- 2.4. Recursive Estimation Algorithms
- 2.5. Optimal Filtering and Least Squares Estimation
-
Chapter 2: Nonlinear Filtering Theory
- 3.1. Introduction to Nonlinear Filtering
- 3.2. The Filtering Problem and Its Challenges
- 3.3. The Kalman Filter and Its Limitations
- 3.4. Nonlinear Models and the Filtering Equations
- 3.5. The Extended Kalman Filter (EKF) and Unscented Kalman Filter (UKF)
-
Chapter 3: Martingales in Filtering Theory
- 4.1. Definition of Martingales
- 4.2. Properties of Martingales and Their Use in Estimation
- 4.3. Applications of Martingales in Filtering
- 4.4. Conditional Expectations and the Filtration Process
- 4.5. The Role of Martingales in Nonlinear Filtering
-
Chapter 4: Stochastic Integrals and Their Applications
- 5.1. Introduction to Stochastic Calculus
- 5.2. Itô’s Lemma and Stochastic Differentials
- 5.3. Stochastic Integrals and Their Properties
- 5.4. Applications of Stochastic Integrals in Filtering
- 5.5. Discrete-Time vs Continuous-Time Stochastic Integrals
-
Chapter 5: Smoothing Techniques in Estimation
- 6.1. Introduction to Smoothing
- 6.2. The Smoothing Problem and Its Algorithms
- 6.3. Optimal Smoothing and Linear Filters
- 6.4. Nonlinear Smoothing Methods
- 6.5. Applications of Smoothing in Time Series and Signal Processing
-
Chapter 6: Advanced Nonlinear Filtering Methods
- 7.1. Particle Filters and Sequential Monte Carlo Methods
- 7.2. Importance Sampling and Resampling Techniques
- 7.3. Nonlinear Filtering in High Dimensions
- 7.4. Filtering with Non-Gaussian Noise
- 7.5. Applications of Advanced Nonlinear Filters in Robotics and Tracking
-
Chapter 7: Numerical Methods in Nonlinear Filtering
- 8.1. Discretization of Stochastic Processes
- 8.2. Numerical Solution Methods for Filtering Equations
- 8.3. Approximation Techniques for Nonlinear Filters
- 8.4. Monte Carlo Simulations for Filtering
- 8.5. Stability and Convergence of Numerical Methods
-
Chapter 8: Applications of Nonlinear Filtering and Smoothing
- 9.1. Tracking and Navigation Systems
- 9.2. Signal Processing and Communication Systems
- 9.3. Robotics and Autonomous Vehicles
- 9.4. Financial and Economic Models
- 9.5. Environmental and Biological Applications
-
Appendices
- A.1. Review of Probability and Statistics
- A.2. Stochastic Process Properties
- A.3. MATLAB and Python for Nonlinear Filtering
- A.4. Glossary of Terms
People also search for Nonlinear Filtering and Smoothing: An Instroduction to Martingales, Stochastic Integrals and Estimation 1st:
smoothing vs filtering
smoothing filter types
nonlinear filtering
filtering and smoothing data
a nonlinear function is